Generative artificial intelligence is booming all around the world. While many governments are working to develop practical policies for the regulation of AI usage, businesses of all sizes are exploring the generative AI possibilities and investing in them:
- According to Deloitte, 41% of organizations plan to use generative AI models in 2024. Deloitte forecasts artificial intelligence and machine learning to drive $4.4 trillion in business value, with the machine learning operations market expanding to $4 billion by 2025.
- A survey by EY shows that 99% of CEOs have plans to invest in generative AI regardless of the complex investment landscape.
- In an outlook by KPMG, 72% of U.S. CEOs say generative AI is a top investment priority for their organizations despite economic uncertainties.
As you want to learn more about generative AI, you should know that, paradoxically, no human will tell you thoroughly how this technology does its magic because the human mind can’t comprehend its tremendous complexity.
Nevertheless, we can explain how generative AI works in general.
To understand what generative AI is, let’s go deeper into computer science and analyze how it differs from traditional artificial intelligence.
The Difference Between AI and Generative AI
AI is well-known as a technology. However, AI is also a discipline that deals with the development and implementation of computer systems that imitate human thinking.
These systems are effective because they can process much more information and at a much faster pace than humans. It’s possible due to machine learning (ML), a branch of AI, or in other words, its ability to repeatedly learn new patterns and rules and use them within a neural network model.
Developers use machine learning to train a model from input data and get output data to resolve tasks, automate workflows, and make predictions.
For solving more complex challenges, there’s deep learning. It’s a branch of ML dealing with models that process large databases, have an ability to learn without human guidance, and have enough knowledge and power to understand the world as an adult human and create new content.
Still challenging to understand the difference? Here’s a little infographic for you:
Traditional AI | Generative AI |
A discipline. | A type of AI. |
Does human tasks more efficiently. | Produces original content from existing content. |
Uses labeled data only. | Uses solely unlabeled data or the mix of labeled and unlabeled data. |
Learns under human supervision from smaller data sets that are similar to each other. | Learns independently or under human supervision from large datasets that are diverse. |
In simple terms, generative AI is the next stage in AI evolution. With the advent of more powerful capabilities, generative AI and traditional AI are now the same thing.
What is Generative AI?
Generative AI is a branch of deep learning and type of artificial intelligence that can create new original content from data it learns from. Generative AI can generate text, images, video, audio, 3D models, and beyond.
A Brief History of Generative AI Models
The starting point for the history of generative AI dates to the first part of the XX century.
In 1936, Alan Turing, one of the fathers of computer science, published his work called Computer Numbers, where he introduced the concept of a computer or, in his words, “universal machine” that could perform any calculation if provided with the proper instructions.
In 1943, Warren S. McCulloch, a neurophysiologist and cybernetician at the University of Illinois at Chicago, and Walter Pitts, a self-taught logician and cognitive psychologist, published their work called A Logical Calculus of the Ideas Imminent in Nervous Activity. In their work, McCulloch and Pills offered the first mathematical model of a neural network.
In 1957, Robert Rosenblatt, a psychologist, published a paper called The Perceptron: A Probabilistic Model for Information Storage and Organization in the Brain, where he introduced one of the earliest machine learning models. The same year, Rosenblatt simulated the model on an IBM 704 computer at Cornell Aeronautical Laboratory.
In 1959, Arthur Samuel, a computer scientist and pioneer in gaming and AI, coined the term “machine learning” when developing his Samuel Checkers-Playing Program that could learn and improve its gameplay against itself.
In the 1960s, Joseph Weizenbaum, a computer scientist at the Massachusetts Institute of Technology (MIT), developed ELIZA, the first ever chatbot and one of the earliest examples of natural language processing (NLP), a subfield of AI aimed at understanding, interpreting, and generating human language.
Between the 1970s and 1990s, many met AI with criticism, hype, and high expectations. Insufficient computational power, the absence of large datasets, and the poor understanding of the human brain and its cognitive functions hampered AI research.
The heyday of generative AI models came in the 1990s when data scientists finally received enough resources to continue AI evolution. For this reason, many models had to wait decades to demonstrate their tremendous potential. Some powerful models, however, appeared only recently.
Main Models + Generative AI Use Cases
After analyzing 63 generative AI use cases across 16 business functions, McKinsey assumed in their report that 75% of the generative AI’s value falls across four areas: software engineering, research & development (R&D), customer operations, and marketing and sales.
Nonetheless, there are many more industries and domains where the benefits of generative AI can completely change the game. Here are the most essential generative AI models (in order of their appearance) and their use cases:
Reinforcement Learning-Based Models
Reinforcement learning (RL) models like to get a reward.
RL models use operant conditioning, a way of learning by rewards and punishments, to teach itself on large datasets of unlabeled data. An RL model takes actions, receives positive or negative feedback, and enhances its performance based on the feedback.
RL models can recognize images, transcribe speech to text, create art, and answer questions. One of the famous RL programs is AlphaGo, that defeated a professional Go player in 2015. Google and Amazon utilize RL to enhance their virtual assistants.
Advantages:
- Lightning-fast data processing and generation. RL models learn quickly, at a large scale, and without explicit programming.
- High flexibility and versatility. RL models learn continuously and can use current knowledge for other environments, tasks, and scenarios.
Applications:
- Gaming. RL models involve AI agents that learn to make human-level and superhuman decisions and imitate more challenging behaviors in video games.
- Autonomous vehicles. Developers use RL models to train self-driving cars and other types of autonomous vehicles to make efficient real-time decisions in complex driving scenarios.
- Robotics. RL models train robots to accomplish complex tasks and enhance their performance in multiple domains.
- Supply chain management. RL models train complex algorithms to analyze large databases to enhance planning, sourcing, manufacturing, production, logistics, transportation, distribution, and other crucial operations.
- Recommendation systems. By learning individual preferences and behaviors, RL models help businesses from different industries, educational institutions, and healthcare organizations provide relevant suggestions.
- Finance. RL models train algorithms to analyze financial markets, enhance investment strategies, and eliminate risks.
Convolutional Neural Networks
Convolutional neural networks (CNNs) revolutionized computer vision, an ability of computers to get information from images, videos, and other data.
In other words, CNNs algorithms can see the world as humans by breaking down content into smaller fragments and recognizing patterns in these fragments. Top technological enterprises like Google, Microsoft, and Meta use CNNs in their multiple products.
CNNs are effective at image classification and segmentation, object detection, style transfer, facial and gesture recognition, and video and text analysis.
Advantages:
- Precise recognition of content. CNNs can recognize different types of content quickly and with high accuracy.
- Smart search. CNNs enable efficient content management automation within large databases due to its ability to quickly and accurately recognize content.
Applications:
- Security. CNNs make surveillance systems more accurate and effective by identifying potential threats and anomalies.
- Self-driving cars. CNNs help autonomous vehicles detect and identify objects and humans in traffic.
- Traffic monitoring. CNNs analyze traffic flows, predict traffic jams, detect accidents, and do other things to enhance road traffic safety.
- Robots. CNNs help robotic systems identify objects within their environments.
- Medical imaging. CNNs analyze medical imaging, such as X-rays, CT scans, and MRIs, more efficiently than humans, which results in more accurate diagnostic outcomes.
- eCommerce. CNNs allows businesses to automate product categorization, enable visual product search, enhance recommendation engines, and develop fraud detection systems.
- Supply chains. CNNs improve supply chain efficiency, profitability, and reliability by predicting demand, enhancing logistic operations, ensuring quality control, and enabling predictive maintenance.
Recurrent Neural Networks
Recurrent neural networks (RNNs) have built-in “memory.” RNNs process sequential and time series data to generate more accurate outputs by memorizing data from previous inputs.
This type of generative AI is effective at machine translation, language modeling, text summarization, sentiment analysis, speech recognition, and time series analysis.
Advantages:
- Advanced predictive analytics. RNNs can understand the context within a particular timeline to make actionable insights and better predictions.
Applications:
- Marketing and sales. With RNNs, online businesses create personalized recommendation systems for customers based on browsing and purchasing history, distinguish customer satisfaction by analyzing customer feedback, and develop chatbots to automate communications.
- Financial services. RNNs allows companies in the financial services sector to forecast trends in the financial market, predict stock prices, identify fraudulent activity patterns, optimize portfolios, enhance risk management, and beyond.
- Pharma. RNNs have enormous potential to design new drugs, enhance drug discovery, accelerate clinical trials, and develop precision medicine.
Generative Adversarial Networks
A generative adversarial network (GAN) is a model where two neural networks compete against each other. The model works on the same reward and punishment principle mentioned above.
The first network, the generator, creates content from existing data. The second network, the discriminator, distinguishes whether content is real or fake. If the generator fools the discriminator, it gets rewarded. The generator’s goal is to fool the discriminator so it can no longer see the difference between real and fake content.
Advantages:
- Realistic reproduction. GANs can create content that is impossible to distinguish from the source or hard to obtain.
- Unique content. GANs can generate original content that has artistic features.
- Training ML data. GANs can produce diverse data to train other ML models and enhance their performance.
Applications:
- Content production. GNNs’ creative possibilities allows a business of any size and from any industry streamline and scale up the production of different types of content.
- Advertising. GNNs have great potential to streamline the production of personalized ads based on the analysis of customer experiences.
- Other creative industries. GNNs may significantly impact art, entertainment, architecture, interior design, fashion, gaming, music, and beyond.
Variational Autoencoders
Variational autoencoders (VAEs) enhance data by compressing it.
VAEs are models that include two neural networks in one. The first network, the encoder, compresses input data, retains only the basic information about it, and reveals the compressed version called the latent space. The second network, the decoder, uses the latent space to reconstruct the original data but in a more efficient form.
Advantages:
- Data efficiency. VAEs can enhance data management within large databases and reduce data storage costs.
- Better decisions and predictions. VAEs can give a deeper understanding of the original data and provide unique insights.
- Enhanced content. VAEs can generate content similar to the original content but unique or even better.
Applications:
- Human resources. Based on advanced data analysis, VAEs can help HR professionals identify the best candidates, predict employee performance, personalize employee training programs, and enhance other operations.
- Telecommunications. VAEs can enhance network performance by predicting network congestion, optimizing network routing, and identifying anomalies. Additionally, telecommunication companies can use VAEs to personalize customer experience.
- Energy. VAEs can help companies in the energy industry optimize energy production and use by identifying inefficiencies, predict energy demand based on historical data, enhance energy trading based on market analysis, and beyond.
- Agriculture. VAEs can help agriculture companies predict crop yield based on historical data, enhance soil quality based on soil data, reduce losses from pests and diseases by analyzing images of crops, and beyond.
Transformer Models
Transformer models revolutionized natural language processing.
Transformer models use the self-attention mechanism to understand context and relationships between different words in sentences more accurately than other models. The most popular transformer model is ChatGPT, where GPT stands as generative pre-trained transformer.
Advantages:
- Parallel processing. Transformer models process multiple parts of the data input sequence simultaneously, which leads to faster training and better performance.
Applications:
- Virtual assistance. Transformers are next-generation chatbots that can aid professionals from a vast range of domains to solve complex challenges.
- Education. With transformer models, students and academic professionals personalize learning experiences, summarize content, translate languages, analyze writings, and assist in many other activities.
- Biomedical research. Transformers’ superhuman analytical power can help researchers find better candidates for clinical trials, simplify access to essential data, better understand role of specific genes in diseases, decipher complex protein folding patterns, and beyond.
- Insurance. Transformers can help insurance companies automate paperwork, predict customer behavior, assess risks more efficiently, identify and prevent fraud, research the market, and beyond.
Diffusion Models
Diffusion models create high-quality images from random noise.
Diffusion models learn how to generate coherent images through repeated applications of the diffusion process: A model starts with a blank canvas, step by step fills it up with a random noise of pixels, and “diffuses” the noise to make an image coherent.
Advantages:
- Realistic images without labeled data. Unlike other generative AI models that can generate high-quality images, diffusion models don’t need labeled data for learning.
Applications:
- Media. Diffusion models can help companies involved in television, gaming, filmmaking, and other types of entertainment generate original content at a large scale.
- Design. Diffusion models can help product companies in various industries design and test prototypes of new products.
- Materials. Diffusion models have great potential to transform material engineering and help companies in this sector design next-generation materials that are more flexible and stronger.
Generative Artificial Intelligence in the Pharmaceutical Industry
Generative AI is a groundbreaking technology that will transform each area of the healthcare, life sciences, and pharmaceutical industries.
In one of our previous articles, we analyzed the impact of AI in life sciences and the current challenges it can eliminate in drug development, clinical trials, precision medicine, and supply chain management.
Here are a few examples of recent transformations caused by generative AI in pharma and healthcare:
Small-Molecule Drug Discovery
Terray Therapeutics, a revolutionary biotechnology company, partnered with NVIDIA to create a platform where generative AI processes hundreds of millions of interactions between small molecules and biological targets daily to accelerate the discovery of new drugs.
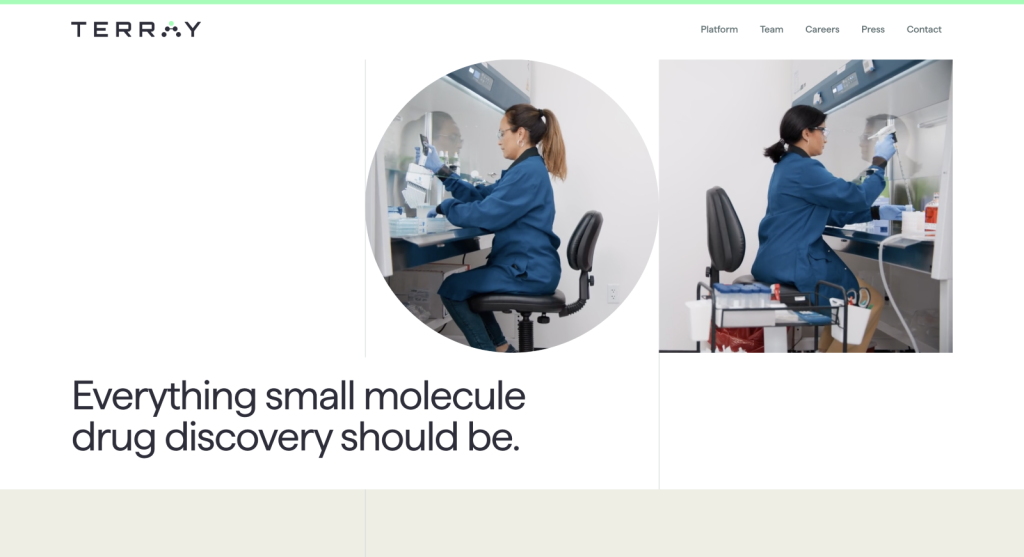
To solve the essential challenges of drug discovery, Terray built COATI, a multimodal VAE model. The model can explore an enormous molecular space at lightning speed due to the computational power unseen before.
Terray used NVIDIA’s cloud solution to reduce the model training period from one week to one day. It allowed the biotech pioneer to design novel enhanced molecules for new drugs even faster.
Smart Healthcare
Care AI is an intelligent care facility platform developed in partnership with the world’s leading tech giants, such as Google, NVIDIA, Samsung, HP, and Lenovo.
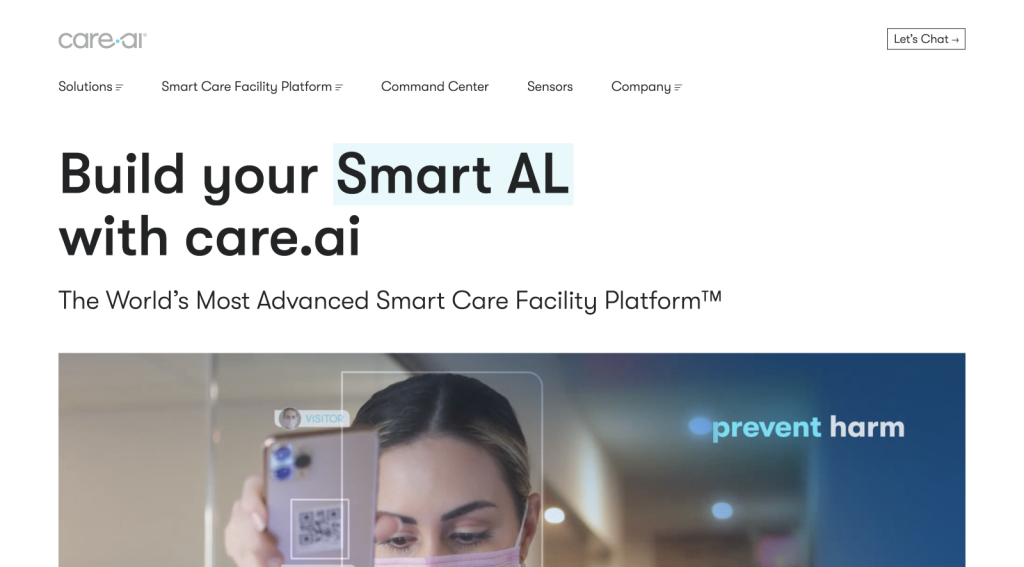
The platform offers cutting-edge AI-assisted solutions seen only in sci-fi movies before, including virtual care, virtual nursing, workflow optimization, patient & protocol monitoring, infection prevention & control, patient & provider experience, compliance & process management, and real-time decision support.
The advantage of Care AI lies in its always-aware ambient sensors that support care teams and significantly enhance their workflows in patient engagement and monitoring.
Mental Health Support
Having surveyed 150,000 adults in 29 countries, Harward Medical School reported that half of the planet’s population will experience a mental health disorder.
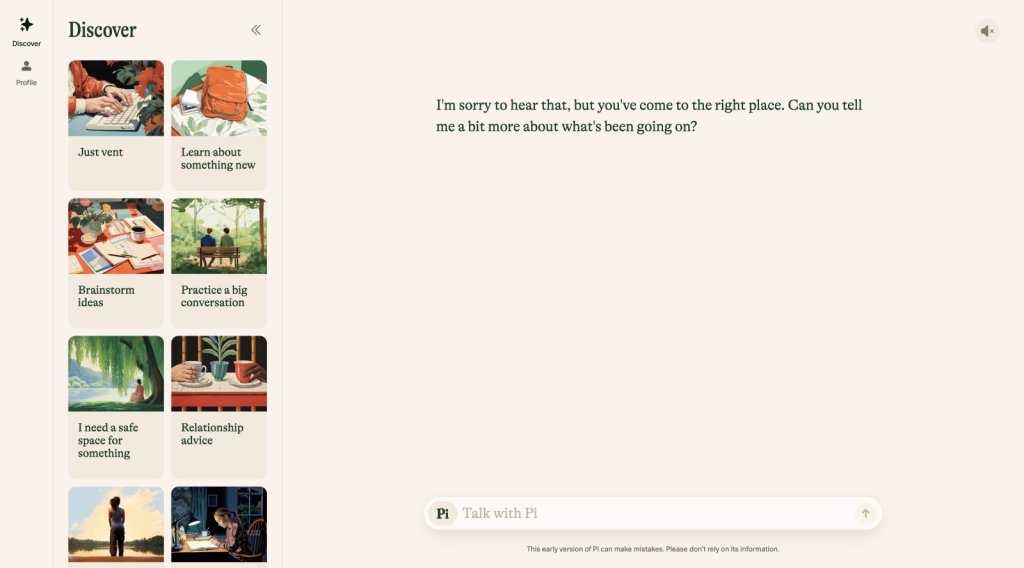
Luckily, generative AI is here to help us with that. Being extraordinarily effective at analyzing human psychology, AI-powered platforms like Wysa and Pi have become a great supplement and alternative to traditional human-to-human talk therapy.
They can assist people facing depression, anxiety, and other mental disorders on an individual level and provide extensive psychological support within complex organizations.
Video Production
As one of the most influential content formats for omnichannel marketing in consumer industries, video is becoming more popular among pharma sales representatives as an effective alternative to eDetailing, the primary format of personalized customer engagement.
eWizard developed an easy-to-use service that allows pharma and life sciences companies and agencies to convert static presentations into interactive videos and create videos from scratch using generative AI.
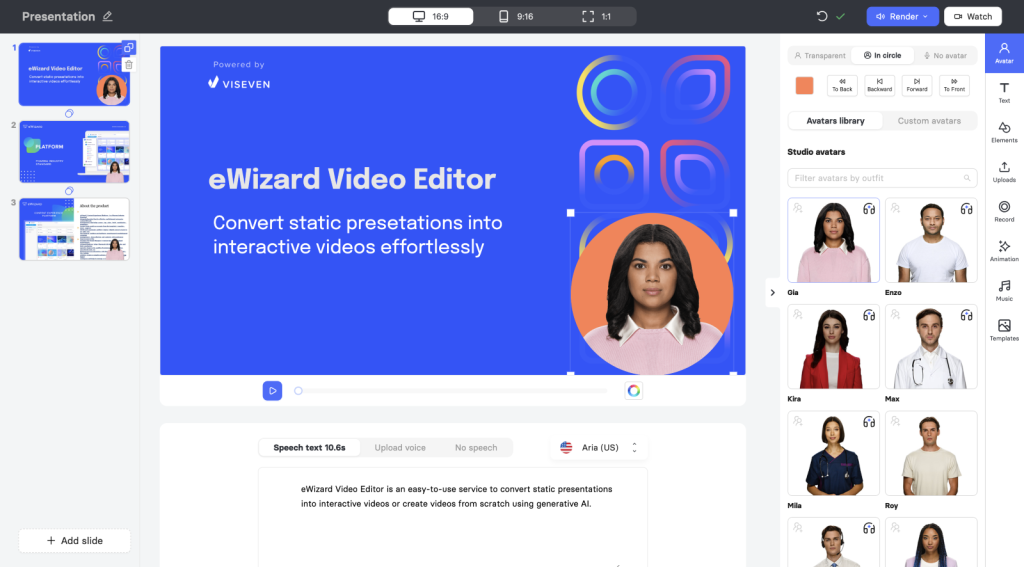
eWizard Video Editor offers all the necessary functionality to streamline video production:
- AI-powered avatars. Enliven video content with a rich collection of avatars that can speak to an audience using text-to-speech generative technology or uploaded audio files.
- Global reach. Create videos for local audiences using AI-powered avatars that speak over 40 languages.
- Full customization. Edit text, images, GIFs, animation, music, videos, and stickers according to your needs and preferences.
- Pre-built templates. Save time and energy on video customization with ready-made slides.
- One-click rendering. Download videos seamlessly in 1080p or 4K to reach any marketing goals.
Have any questions? Request a demo or talk to an expert now, and we’ll get back to you shortly.